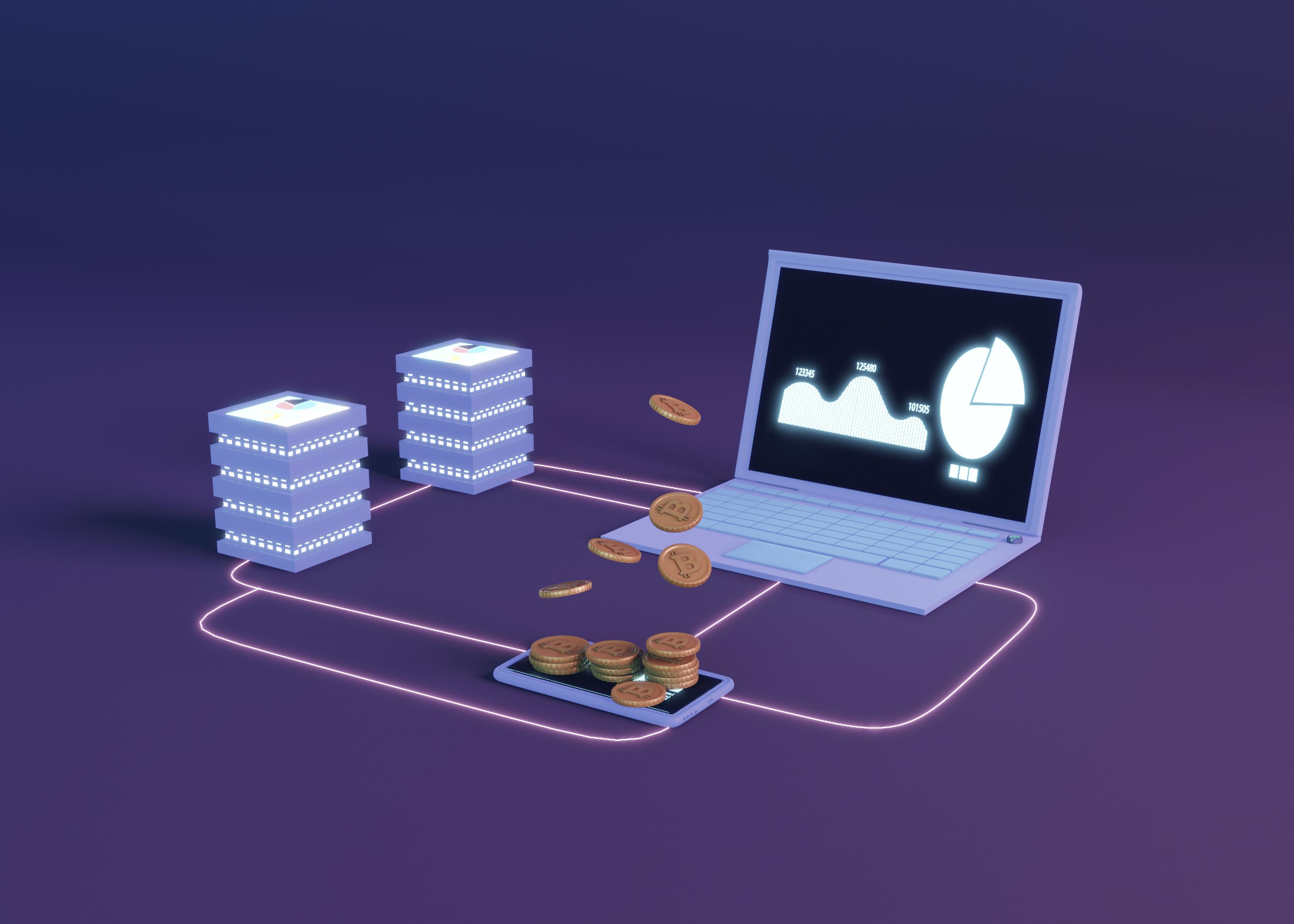
How Can Machine Learning Protect Your FinTech Solution From Fraudulent Attacks?
May 21, 2024
The banking and fintech sector faces an ongoing challenge from individuals attempting to engage in fraudulent activities. The conventional methods employed to identify fraud are grappling with the evolving tactics employed by these individuals. To counter this issue, financial institutions and banks are embracing AI-driven solutions.
These innovative solutions harness the power of machine learning to efficiently identify, surveil, and thwart fraudulent actions. In the forthcoming discussion, we will delve into how machine learning serves as a protective shield for fintech applications against malicious assaults.
What is a Financial Fraud?
Financial fraud encompasses various fraudulent activities that manipulate financial tools, like credit cards, bank holdings, or investment portfolios, with the illicit intention of acquiring funds. This multifaceted problem manifests through diverse avenues, spanning from identity appropriation and digital swindles to intricate stratagems that entangle numerous participants.
Financial fraud can generally be classified into four main groups:
Carding
Carding is an illegal activity where individuals, known as "carders," use stolen credit card information or engage in fraudulent methods to make unauthorized purchases or financial transactions. This can involve using stolen credit card details to buy goods online, transfer money, or obtain other financial benefits without the cardholder's consent.
Phishing
Phishing is a cybercrime where scammers impersonate trusted sources to trick individuals into revealing sensitive information, like passwords or credit card details, through fake emails or websites. This stolen information is then used for fraudulent purposes. It's crucial to be cautious and verify online messages and websites to protect personal data.
Maleficient software
Malicious Software Financial Fraud is a form of cybercrime where malicious software, or malware, is used to illegally access and exploit financial information for monetary gain. This typically involves stealing sensitive financial data, like credit card details, bank account information, or personal identification, and using it for fraudulent financial activities, including unauthorized transactions and identity theft. This type of fraud is executed through tactics like phishing emails or compromised software.
Mobile fraud
Mobile financial fraud refers to any deceptive or illegal activity conducted through mobile devices, such as smartphones or tablets, with the intent to steal money, personal information, or access financial accounts. It includes various fraudulent practices, such as phishing, identity theft, fake mobile apps, and unauthorized transactions, that exploit vulnerabilities in mobile technology and apps to defraud individuals or organizations.
Why is Fraud Detection Vital in Fintech?
Ensuring the integrity of financial transactions holds immense significance within the fintech realm. This significance is underscored by the sheer volume and intricate nature of electronic transactions that transpire daily.
As technology continues its rapid evolution, criminal elements have likewise honed their skills, demonstrating heightened sophistication in circumventing established methodologies and data analysis protocols aimed at deterring fraudulent activities.
Consequently, financial service providers find themselves bearing legal responsibility for any resulting losses stemming from fraudulent acts. This heightened legal responsibility inevitably inflates the operational costs associated with conducting business in this sector.
In recent years, there has been a substantial surge in the sheer volume of data accessible to businesses, posing a significant challenge in detecting fraudulent activities within the fintech sector. To address this issue, numerous enterprises have initiated the deployment of machine learning-driven systems utilizing advanced algorithms like deep learning for payment fraud detection.
This approach reveals concealed instances of fraud by unveiling intricate correlations and associations present within extensive datasets. It also empowers companies to unearth elusive insights that often remain obscured in the midst of data noise.
In essence, the utilization of such technologies empowers businesses to identify and mitigate fraud risks proactively, safeguarding users from potential victimization, all the while optimizing cost-efficiency in their fraud prevention efforts.
To safeguard fintech applications from the perils of fraudulent activities, it becomes imperative to harness the capabilities of machine learning algorithms. Machine learning, an artificial intelligence methodology, serves the purpose of foreseeing future occurrences and uncovering underlying patterns. This technology empowers analysts to discern anomalies within the dataset, facilitating the prompt and precise identification of deceitful transactions.
The Work Principles of Anti-Fraud Systems
Anti-fraud measures safeguard financial technology applications against illicit intrusions. These protective mechanisms leverage machine learning algorithms to scrutinize user conduct data. Factors such as login attempts, payment track records, and transaction particulars are all factored into the analysis. Through a comprehensive examination of this information, the system can identify potentially suspicious actions and promptly alert users to potential fraudulent activities.
Functioning principle
Anti-fraud systems operate by scrutinizing user behavior data, such as login attempts and payment records, in order to uncover potential fraudulent activities. Utilizing machine learning algorithms, these systems cross-reference this data with well-established patterns to pinpoint any deviations that could signify potential fraud. Moreover, augmenting security measures, two-factor authentication necessitates users to furnish dual forms of identification, further bolstering protection.
Data analysis
Machine learning possesses the ability to scrutinize customer transaction records, encompassing details such as transaction category, monetary value, and recurrence. This data serves as a valuable tool for uncovering irregularities in customer conduct that could potentially signal fraudulent actions. Enterprises can leverage machine learning to pinpoint and mitigate fraudulent behaviors in advance of them evolving into significant issues.
Architecture
The structural design of the anti-fraud system may encompass the following elements:
- - Data preprocessing and collection. The first step entails the collection of information from a variety of outlets, such as client databases, financial records, card transactions, ATM withdrawals, and other pertinent sources. Following this, the acquired data must undergo a preparatory phase to ensure its purity and appropriateness for deployment in machine learning algorithms.
- - Machine learning models development and training. Once the data has been appropriately processed, it becomes amenable for the development of models aimed at detecting irregularities in financial transactions. Various ML algorithms, including Neural Networks, Decision Trees, and Support Vector Machines (SVM), find utility in fraud detection. To ensure their effectiveness in classifying unfamiliar datasets, these models necessitate training with annotated datasets containing instances of fraudulent transactions.
- - Model implementation and monitoring. After the training process is complete, the model must be implemented in a real-world operational setting. Within this context, ongoing surveillance of its performance becomes essential, aimed at sustaining a sufficiently high level of precision in identifying potentially suspicious actions, all the while minimizing the occurrence of erroneous alerts or false positives.
- - Model over time support and maintenance. As fresh forms of fraudulent activities surface, it becomes imperative to conduct retraining exercises on the model by incorporating the latest datasets. This practice is crucial to maintain the continued efficacy of the model in identifying and countering emerging fraud risks.
Machine Learning and Fraud Detection: How to Compose Them?
A machine learning system designed for detecting fraudulent activities initiates its process by gathering and categorizing data. The primary objective is to furnish machine learning models with information capable of yielding dependable predictions. As such, a diverse array of data sources is harnessed to construct a comprehensive dataset. This compilation encompasses transactional records, customer profiles, and pertinent data elements.
Once the dataset has been meticulously prepared, the subsequent phase entails the integration of this dataset into algorithms intended for the purpose of training the model to identify potential fraudulent activities. The specific approach utilized depends on the nature of the system under development, with the possibility of employing either supervised or unsupervised methodologies to uncover irregular patterns and behaviors.
Various classification techniques, such as logistic regression, decision trees, or clustering algorithms, are systematically applied to categorize transactions and activities based on their associated risk levels. This process equips the machine learning system with the capability to promptly and accurately pinpoint entities or activities displaying fraudulent characteristics, often even before they occur in real-time scenarios.
As new data continuously streams in from diverse sources throughout networks, the machine learning model consistently enhances its accuracy by refining its rules through the inclusion of fresh examples extracted from recent activities.
Ways to Prevent Fraud Using Machine Learning
Before even thinking about how to create a financial app, it’s crucial to find ways to safeguard it properly on the shore. Machine learning is revolutionizing the way Fintech enterprises identify real-time fraudulent activities. Outdated methods, such as rule-based systems, are gradually giving way to ML algorithms, which scrutinize user actions for enhanced fraud detection. Predictive analytics play a pivotal role in enabling ML to forecast user behavior with precision. Additionally, unsupervised learning combs through extensive datasets to uncover irregularities that often elude human detection.
ML techniques have the potential to significantly diminish the occurrence of erroneous fraud alerts. Conventional approaches frequently yield inaccurate alarms, leading to undesirable interruptions and operational inefficiencies. Machine learning, however, mitigates these false positives, resulting in a more precise identification of potential security risks, ultimately bolstering the integrity of business safeguarding measures. Given its ability to drive down expenses and amplify operational effectiveness, financial technology (Fintech) enterprises have grown increasingly interested in leveraging machine learning to fortify their security and fraud prevention strategies.
Supervised learning
Supervised learning stands as a branch of machine learning designed for the purpose of discerning fraudulent activities within transactional data. Historically, it was employed prior to the era of neural networks for the identification of deceitful transactions.
Engineers of the time employed statistical techniques such as clustering, attribute selection algorithms, decision trees, and regression to forecast instances of fraud. These models, though sizeable, were relatively straightforward and lacked intricate connections.
The application of neural networks in machine learning posed certain challenges for engineers, chiefly due to the constraints imposed by limited computational power and the state of scientific knowledge at the time. This resulted in the utilization of modest datasets and the development of rudimentary models for fraud prediction.
Nonetheless, progress in computational capabilities and scientific research has substantially enhanced the efficacy of supervised learning, rendering it more proficient than deep learning algorithms. These refined models empower businesses to construct more precise fraud detection software, thereby reducing both false positives and false negatives.
Unsupervised learning
Unsupervised learning represents a category of machine learning algorithms with the primary goal of enhancing the security of FinTech applications against deceptive assaults. Unlike supervised learning, this approach doesn't rely on labeled data; instead, it leverages statistical analysis as its foundation. Its core function involves scrutinizing extensive datasets to unearth underlying patterns, with a specific focus on pinpointing irregularities within these data collections.
These irregularities often serve as potential indicators of fraudulent activities, which can be exceptionally intricate for human observers to discern due to their intricate interdependencies among various variables. Nevertheless, unsupervised learning algorithms excel in efficiently and accurately uncovering these intricate correlations, thereby assisting organizations in fortifying their applications against fraudulent incursions.
The identification of outliers within datasets plays a pivotal role in detecting malevolent actors seeking to exploit vulnerabilities. These outliers serve as valuable cues in both forecasting and preempting fraudulent behaviors. Furthermore, unsupervised learning extends its capabilities to identify fraudulent individuals already embedded within the system by unveiling suspicious patterns hidden within the data. This proactive approach aids in curtailing the detrimental impact caused by fraudulent activities.
Reinforcement learning
Reinforcement learning has gained significant popularity among fraud detection systems aiming to outwit their adversaries. This method empowers these systems to continuously adapt to the evolving tactics employed by fraudsters as fresh data continuously enriches their learning processes. Consequently, this ensures that the model remains current with emerging technologies and strategies employed by malicious actors, all while maintaining robust resistance against attempts to incapacitate it.
The application of reinforcement learning also offers a guarantee of improved detection accuracy over time. Even in their initial testing stages, these models often achieve accuracy levels nearing 90%. Subsequently, as more data becomes integrated into the learning process, these rates can be sustained and even elevated.
This implies that within just a few years of being in operational use, the model could attain near-perfect accuracy scores, effectively adapting to nuanced shifts in fraudulent activities. Such models create an environment that virtually eliminates opportunities for fraudsters to exploit vulnerabilities without being apprehended.
Conclusion
Furthermore, contemporary anti-fraud systems must adhere to precise benchmarks to ensure their efficacy. The machine learning algorithms employed should possess the capability to accurately identify both familiar and unfamiliar instances of fraudulent behavior. Striking a delicate equilibrium between minimizing false positives and safeguarding consumer interests is crucial.
Additionally, automated decision-making processes need to maintain transparency by furnishing customers with lucid rationales for flagging their activities as suspicious or unacceptable. Lastly, organizations ought to give paramount importance to safeguarding user privacy, ensuring that only pertinent data is gathered and employed responsibly for the purposes of thwarting fraud.