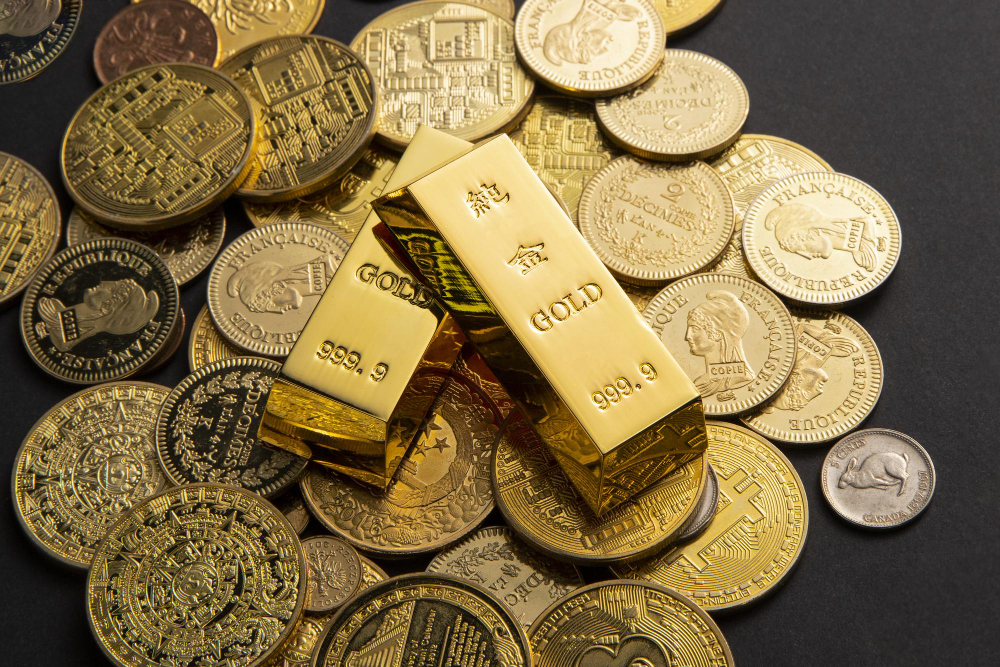
Gold, often regarded as a symbol of wealth and security, has always been a crucial investment for traders and individuals alike. However, predicting the price of gold has been a formidable challenge, due to its sensitivity to a variety of factors like economic stability, currency fluctuations, and geopolitical events. With the advent of advanced technologies, machine learning has emerged as a game-changer, making gold price prediction more accurate and timely than ever before.
What is Machine Learning?
To understand how machine learning can predict gold prices, it’s essential to grasp the basics of what machine learning is. Machine learning is a subset of artificial intelligence (AI) that focuses on developing algorithms that allow computers to learn from and make decisions based on data. By processing vast amounts of historical data, machine learning models can identify hidden patterns and relationships, making predictions without needing explicit programming for each scenario.
This capability makes machine learning ideal for complex fields like financial markets, where countless variables influence outcomes. For gold price prediction, machine learning uses various models to analyze a wide array of factors that impact gold prices, offering more refined and actionable forecasts.
Why is Gold Price Prediction So Challenging?
Gold prices are affected by multiple, often interconnected factors, making their prediction particularly difficult. Here are some of the key drivers of gold prices:
- Global Economic Conditions: During economic downturns or periods of uncertainty, gold is often viewed as a safe-haven asset, driving up demand and consequently, its price. Similarly, during economic booms, demand for gold may decline as investors look for higher returns elsewhere.
- Interest Rates: When interest rates are low, the opportunity cost of holding non-yielding assets like gold decreases, making gold a more attractive investment. This tends to drive gold prices higher.
- Currency Movements: Gold prices often move inversely to the U.S. dollar. A weakening dollar generally leads to an increase in gold prices as it becomes cheaper for investors holding other currencies.
- Inflation Rates: Gold is traditionally seen as a hedge against inflation. When inflation rises, gold prices tend to follow, as investors seek to preserve the purchasing power of their assets.
- Geopolitical Events: Wars, natural disasters, and political instability can have immediate and significant impacts on gold prices, as these events create market uncertainty.
These factors, combined with others such as supply-demand dynamics and trade policies, make manual prediction of gold prices extremely challenging. This is where machine learning comes in, automating the analysis of these complex factors to provide more reliable price forecasts.
How Does Machine Learning Work in Gold Price Prediction?
The process of predicting gold prices using machine learning involves several steps, each playing a crucial role in enhancing the accuracy of the final predictions. Let's break down how these models operate:
1. Data Collection and Preprocessing
The foundation of any machine learning model is data. For gold price prediction, historical data is gathered from multiple sources, including past gold prices, inflation rates, interest rates, stock market indices, currency exchange rates, and geopolitical events. This raw data is then preprocessed—cleaned and structured—to ensure that only relevant, high-quality information is used.
Data preprocessing often involves removing outliers, filling in missing values, and normalizing or scaling the data so that the machine learning algorithms can easily interpret it. This step ensures that the model does not make biased predictions based on faulty or incomplete data.
2. Feature Selection
Not all variables affect gold prices equally. For example, while interest rates and inflation have a strong correlation with gold prices, other factors may have a weaker or negligible impact. Therefore, feature selection is crucial in narrowing down the most influential variables that will be used in the prediction model.
Some common features used in gold price prediction models include:
- Historical gold price data
- Interest rates
- Inflation rates
- Stock market indices
- Global economic indicators
- Currency exchange rates, particularly the U.S. dollar
Selecting the right features improves the accuracy of the machine learning model and ensures it remains efficient without unnecessary complexity.
3. Training the Model
Once the features have been selected, the machine learning model is trained. This involves using a portion of the preprocessed data to "teach" the algorithm how different factors have historically influenced gold prices. The machine learning model learns from this data by identifying patterns and relationships between the variables.
Several types of machine learning algorithms can be used for gold price prediction, including:
- Linear Regression: This is a simple yet powerful tool for identifying the relationship between dependent and independent variables. In the case of gold prices, it might track how inflation or interest rates affect prices over time.
- Support Vector Machines (SVM): SVMs are powerful algorithms that can model complex relationships in data, making them particularly useful for gold price prediction where many variables interact.
- Neural Networks: These are advanced machine learning models that mimic the functioning of the human brain. Neural networks excel at identifying non-linear relationships and can produce highly accurate predictions.
4. Model Testing and Validation
After the model is trained, it needs to be validated to ensure that its predictions are accurate. This involves testing the model on a separate set of data (often called the test dataset) that it hasn’t seen before. By comparing the model’s predictions with the actual gold prices from the test data, developers can assess its performance.
Common validation techniques include cross-validation and backtesting, where the model is tested on historical data to see how well it would have predicted past price movements.
5. Model Optimization
If the model’s predictions are not accurate enough, developers can fine-tune it through optimization techniques. This might involve adjusting the features, modifying the algorithms, or retraining the model on new or more diverse datasets. The goal of optimization is to continually refine the model so that its future predictions become increasingly accurate.
Types of Machine Learning Models for Gold Price Prediction
Different types of machine learning models are used for gold price prediction, depending on the complexity and type of data:
1. Supervised Learning Models
These models are trained using labeled datasets, where both the input features and the output (gold price) are known. The model learns the relationship between these features and the price, making future predictions based on this learned knowledge.
2. Unsupervised Learning Models
Unsupervised learning models are used when the data does not have labeled outputs. These models identify patterns and clusters in the data, offering insights that can indirectly influence gold price predictions. While not commonly used directly for price prediction, unsupervised learning can still provide valuable supplementary information.
3. Reinforcement Learning Models
Reinforcement learning is another approach where the model learns through trial and error. The model continuously refines its predictions based on real-time feedback from the market, making it highly adaptable to new data.
Benefits of Using Machine Learning for Gold Price Prediction
Machine learning offers several advantages for predicting gold prices:
1. Improved Accuracy
Machine learning models can process large volumes of data from diverse sources, analyzing complex relationships between variables. This allows for more accurate predictions than traditional methods.
2. Speed
Unlike traditional financial analysis methods, which can be time-consuming, machine learning models can generate predictions in real-time. This is particularly beneficial for traders who need to make quick decisions.
3. Adaptability
Machine learning models are highly adaptable and can learn from new data. As more data becomes available, the models continue to evolve, improving their accuracy and relevance.
Challenges of Machine Learning in Gold Price Prediction
While machine learning offers numerous benefits, it is not without challenges:
- Data Quality: Machine learning models rely heavily on data quality. Poor or incomplete data can result in inaccurate predictions, which can be detrimental for investors.
- Overfitting: If a model becomes too focused on the historical data it was trained on, it may struggle to make accurate future predictions, especially in volatile markets like gold.
- Market Volatility: Gold prices are highly sensitive to sudden economic or geopolitical shifts, and even the most advanced models can struggle to predict such short-term movements.
Conclusion
Predicting gold prices has always been a complex challenge due to the numerous factors involved. However, machine learning is revolutionizing this process, providing more accurate and timely forecasts than ever before. By analyzing vast amounts of data and identifying hidden patterns, machine learning models offer traders and investors a powerful tool to navigate the volatile gold market.
As machine learning continues to evolve, its applications in financial markets will only become more refined, allowing for even better predictions. For those looking to stay ahead in the gold market, leveraging machine learning models can offer a significant competitive edge.
Source: https://diigo.com/0xw9ro